Interpreting Machine Learning for Business Strategy: The Role of Managers
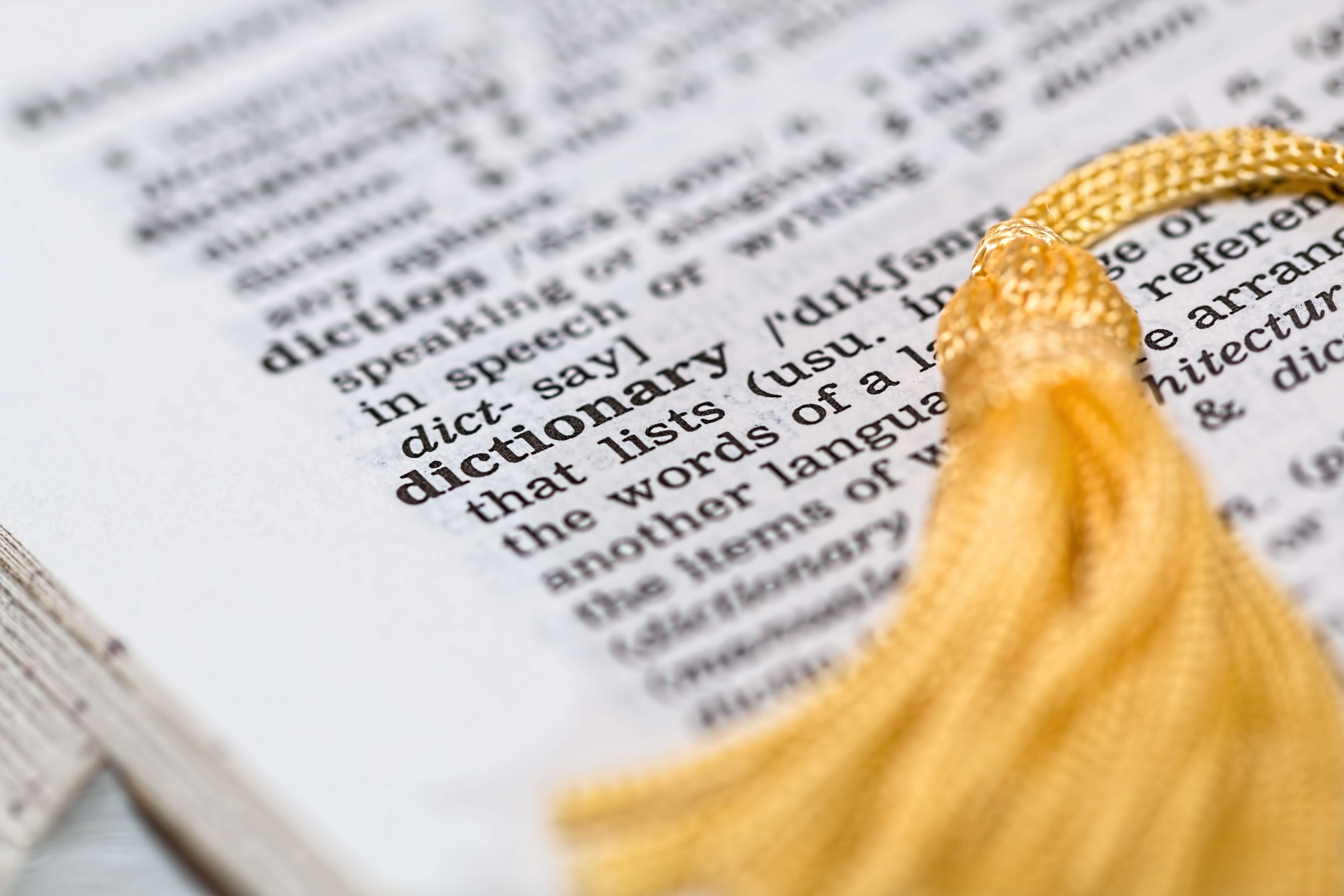
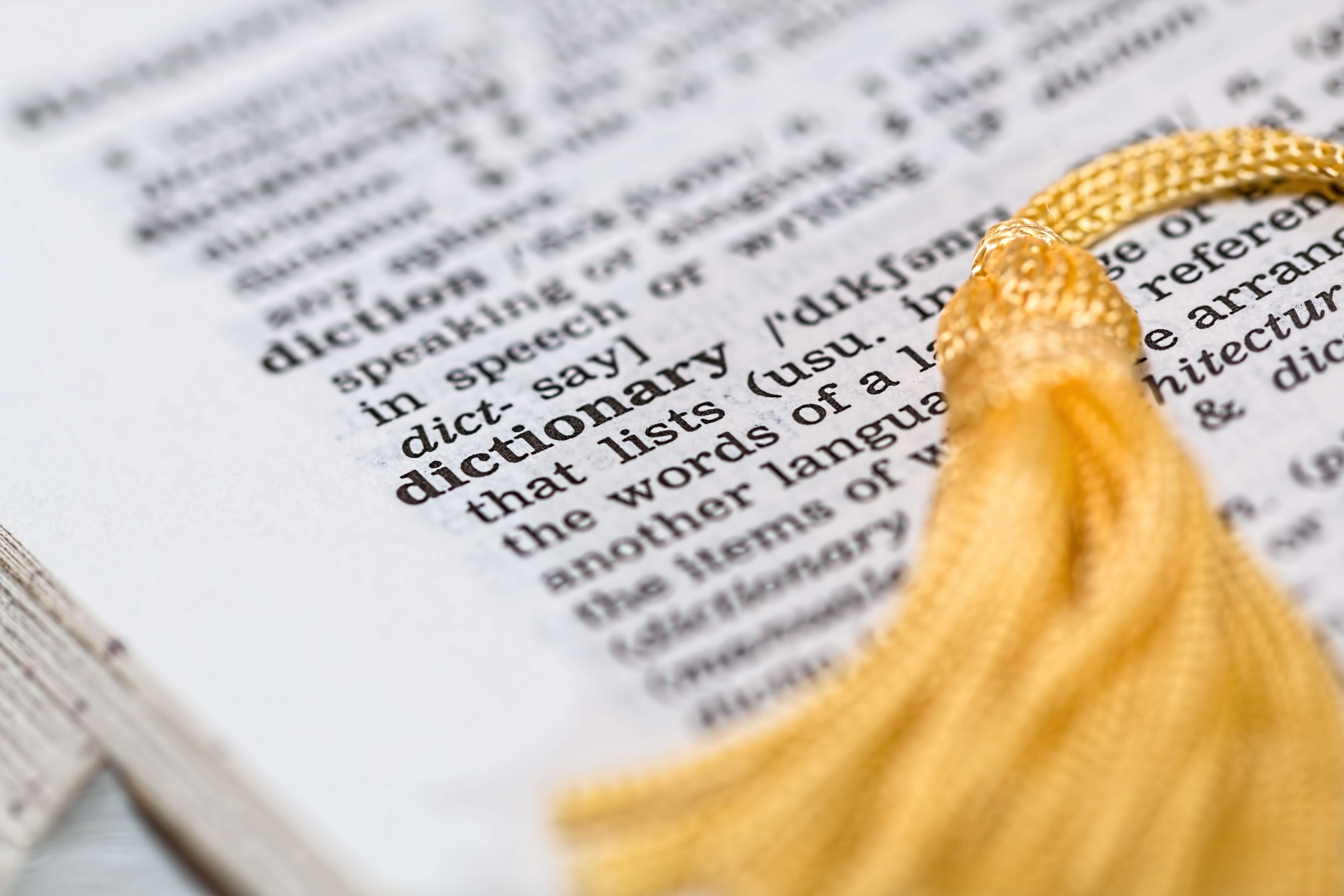
Real world data is dirty. It comes with missing values and is often labelled in a confusing, made up language. Data in its raw form will not help inform business strategy. Even if it is processed and cleaned, the elements of a dataset that impact ML models may not allow management to take action and achieve their goals. When initiating an ML project, managers need to be involved in the early stages, provide their industry expertise, and assist the data scientist in feature engineering.
According to Dr. Jason Brownlee, “Feature engineering is the process of transforming raw data into features that better represent the underlying problem to the predictive models, resulting in improved model accuracy on unseen data.” In other words, feature engineering is the process of taking meaningless data points and transforming them into the insights that can improve the model. These insights are also what management needs to inform business strategy. By involving management in feature engineering, raw data can be transformed into a rich set of features that can dramatically affect the way that a business crafts its strategy. To provide some clarity on this, let’s imagine a scenario in which an international car company wants to improve customer loyalty.
The “Cool Car Company” defines loyalty as customers who dispose of their old Cool Car and get a newer Cool Car model. They have millions of different data points on their customers and want to find out which ones are indicative of customer loyalty. They will then increase their marketing efforts on the customers who are identified as likely to be loyal and nudge them towards a new vehicle.
The data scientist begins working on the data herself. She extracts the data categories that are most correlated with customers that are likely to be loyal and ends up with the following:
- Lease Customer
- Contract Holders
- 5 Year Term
She presents these findings to Cool Car Company’s leadership and is met with a lot of confusion. These data points seem to say that lease customers who are also the contract holders and have terms of 5-years are likely to be loyal. That's a mouthful, and in no way can inform business strategy. The company is targeting existing Cool Car customers and they cannot expect to switch them, after the fact, into lease contracts with 5-year terms. She responds to their confusion with a suggestion to engage a manager who is an expert in the car industry and involve them in a feature engineering exercise.
The data scientist and manager sit down to discuss how they can extract actionable insights from the raw data. The manager explains that when customers are in the dealership and there are new models are on display, customers are much more likely to upgrade to the new model. He’s also noticed that customers who pay less out of pocket for repair and maintenance are more likely to purchase another vehicle with the company.
With this in mind, the data scientist goes back to the raw dataset. She inspects some of the data features that align with what the manager has said and engineers new categories using the raw data:
- Car Serviced at the dealership 5+ times
- Extended Warranty Coverage
- Wear and Tear Coverage
She then runs her model using these features and finds they predict customer loyalty at a high rate. Now that the model is trained (on a smaller subset of data), the data scientist applies it to the full customer dataset. She returns to management with a prediction of whether each existing customer will be loyal or not.
With these results, management can begin crafting strategy to make service visits, extended warranty, and wear and tear insurance more affordable. They could offer discounts and market these offerings more effectively to customers. Then, they could monitor the effect these tactics have on loyalty and measure the impact to their bottom line. By involving a manager with industry expertise, the data scientist uncovered an intuitive roadmap the company can use to achieve their goals. This approach allows companies to harness the power of ML and enjoy exceptional results driven by this technology.
Chapters
Trip Images
Want to stay in the loop?

Mitchell Johnstone
Director of Strategy
Mitch is a Strategic AI leader with 7+ years of transforming businesses through high-impact AI/ML projects. He combines deep technical acumen with business strategy, exemplified in roles spanning AI product management to entrepreneurial ventures. His portfolio includes proven success in driving product development, leading cross-functional teams, and navigating complex enterprise software landscapes.